PhD opportunities
EPCC offers the opportunity to study for a PhD in areas related to High Performance Computing (HPC), Computational Science, Data Engineering, Data Science, and Software Sustainability.
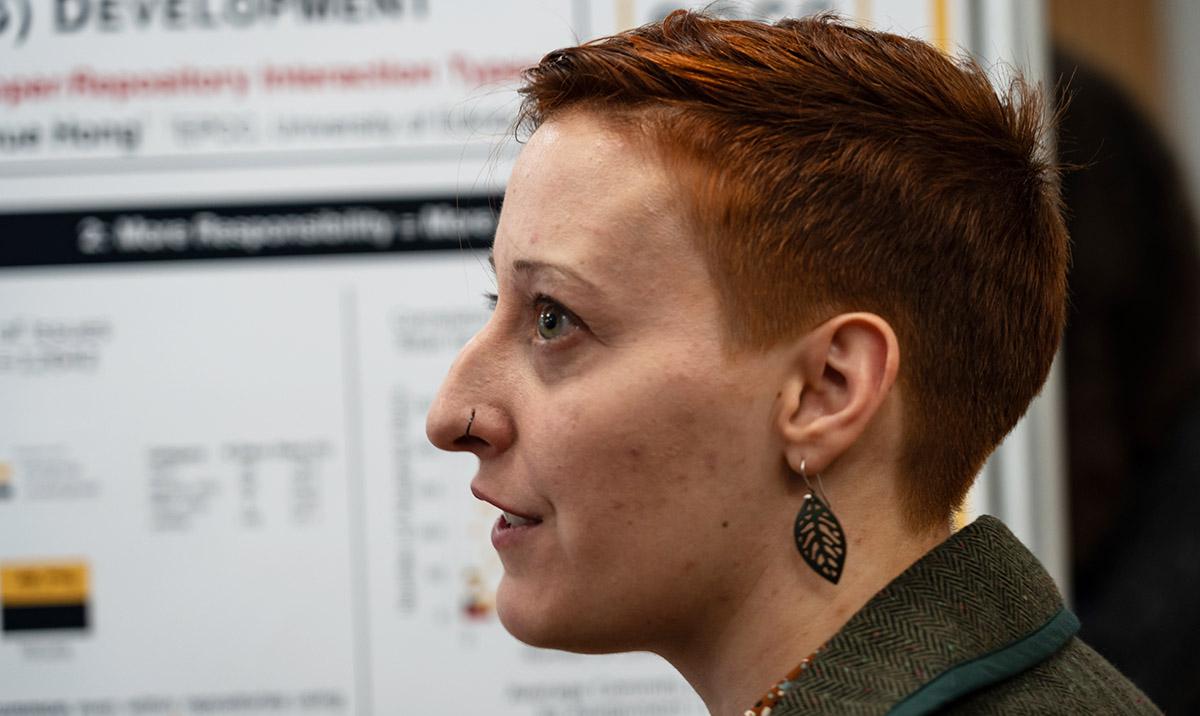
How to apply
Applications are made via the 'Apply' link on the University of Edinburgh degree finder. Once registered for the Applicant Hub, you can track your application there. There is more information on the degree finder page, but your application will need to contain a research proposal, the basis of which will likely be one of the projects listed below or one of your own creation (see below for more information).
Application Process
We would usually expect applicants to discuss their application with a potential supervisor before applying either by e-mailing the supervisor directly or via phd@epcc.ed.ac.uk.
Once your application has been received and all relevant documents have been checked, applicants will be invited to interview (often remote, but in-person is also possible) with the potential supervisor and at least one other member of staff.
Potential research topics
Please contact phd@epcc.ed.ac.uk for more information on any projects listed below. Projects are grouped by general subject area.
HPC
Obtaining performance portability via Domain Specific Languages (DSLs) and MLIR
Dr Nick Brown, Dr Tobias Grosser (School of Informatics)
Supporting heterogeneous architectures in ASiMoV-CCS
Dr Michèle Weiland
Geometric Deep Learning for Particle-Based Computational Fluid Dynamics
Dr Joseph O’Connor
Artificial Intelligence (AI)
Scalable Dynamic Deployment of Data-Flow Applications in Heterogeneous Environments through AI-Driven Optimization
Dr Rosa Filgueira
Ontology-Guided Explainable AI for Enhancing Software Repository Comprehension
Dr Rosa Filgueira
Intelligent Tutor System (ITS) for Children with Autism using Generative AI
Dr Charaka Palansuriya
Advancing Software Understanding and Composition through Machine Learning-driven Semantic Analysis
Dr Rosa Filgueira
Software Sustainability
Quantification of the environmental footprint of research software to help the community achieve Net Zero
Dr Kirsty Pringle
ExaGEO PhD Student Projects
The Exascale computing for Earth, Environmental, and Sustainability Solutions consortium ( ExaGEO) will train the next generation of Earth and environmental scientists to harness the power of Exascale computing. It is co-led by EPCC, researchers from the University of Glasgow, Lancaster University’s Centre of Excellence in Environmental Data Sciences, and partners from industry and government. See the ExaGEO website for currently available projects.
Proposing a project
We also welcome proposals in one of EPCC's areas of research interest. Before applying we would expect an applicant to contact a potential supervisor to discuss a draft proposal. Please contact phd@epcc.ed.ac.uk if you are unsure who would be the best potential supervisor to contact.
The University has guidance available on writing a research proposal.
Partnership with industry
Like EPCC's Research Collaborations with industry, a collaborative PhD project with an industrial partner is possible. There are various ways this can work so please contact phd@epcc.ed.ac.uk and/or any particular potential supervisor if you would like to discuss options.