AI-training trial yields impressive results with EPCC's Cerebras CS-2
18 January 2024
smartR AI™ and EPCC are working together on an AI trial using the Cerebras CS-2 Wafer-Scale Engine (WSE) system. The results so far have shown a dramatic reduction in AI training times.
Here at EPCC, we believe the impressive results from smartR AI described below give clear confirmation that the Cerebras CS-2 is a game-changer for training large language models. The Cerebras team has recently developed new upgrades for the system which we expect will enable training times to be reduced even further, and we look forward to sharing these benefits with our partners.
Matthew Malek, an engineer at smartR AI, explains the remarkable results achieved so far.
smartR AI is a Scottish-based consultancy specialising in Natural Language Processing (NLP) applications of AI. The collaboration with EPCC, the UK's leading centre of supercomputing and data science expertise, originated through a need for comparing the performance of EPCC’s Wafer-Scale Engine CS-2 chip with an NVIDIA RTX-3090 graphical processing unit (GPU) in the context of training and fine-tuning large language models (LLMs). To address these issues, we have conducted a comparative analysis of the training convergence time on both processing units.
Hardware comparison
We conducted a comparison between two advanced hardware setups designed for optimized parallel computation.
First we examined the Cerebras Wafer-Scale Engine CS-2 chip, notable for its colossal compute core scale with 850,000 AI-optimized cores. This chip addresses deep learning bottlenecks by efficiently utilising its cores and 40 GB of on-chip memory, boasting an exceptional 20 PB/s memory bandwidth. Conversely, the smartR AI Alchemist server employs the NVIDIA RTX 3090, positioning it as a promising solution for on-premise fine-tuning of LLMs.
"Staggering" results
To ensure fairness, we aligned CUDA and WSE core counts for direct comparison of training loss conversion performance. This head-to-head analysis sheds light on parallel computation possibilities, impacting the evolution of LLMs and deep learning applications. The results are staggering, with our GPT-2 model pre-training becoming optimised five times faster.
In our comparison experiment, we conducted pre-training on a version of the GPT-2 model. As the original OpenAI GPT2 paper uses, we employed an open version of WebText, OpenWebText, which is an open-source recreation of the WebText corpus. The text is web content extracted from URLs shared on Reddit with at least three upvotes (38GB).
We used a Byte-Pair Encoding (BPE) tokenizer with a vocabulary size of 50,000. However, our GPU's hardware limitations led us to keep the context size of this dataset at 512 units. This choice helped us work within our GPU's capabilities while still serving the purpose effectively.
In a significant research effort, we conducted a comparison by pre-training a smaller version of the GPT-2 model. We engaged in a comprehensive training process that spanned 150,000 training steps, culminating in the point where training loss was converged.
This specific version of the GPT-2 model boasts 117 million parameters and a context size of 512. Using a Byte-Pair Encoding (BPE) tokenizer with a vocabulary of 50,000 words, we maintained precision by using full float32 for model weights, which required a memory allocation of 512 megabytes. Due to the hardware limits in our GPU, we could not create a model with context size longer than 1024, which modern LLMs have.
Throughout the training process, we utilized a batch size of 16 and implemented the AdamW optimization algorithm with a learning rate of 2.8E-4, alongside a learning rate scheduler.
Thus far, running the sample models smartR AI has managed to train a model from scratch in nearly one hour on the EPCC system with the Cerebras CS-2 chip, compared to the massive 10 hours that it took to complete on smartR AI's own internal system with a NVIDIA RTX 3090 GPU. The company’s engineers working on this project are quite certain they will be able to utilise more resources on the EPCC’s Cerebras system and so speed it up even more.
The following graphic shows the results of GPT training on EPCC's CS-2 server compared to internal servers.
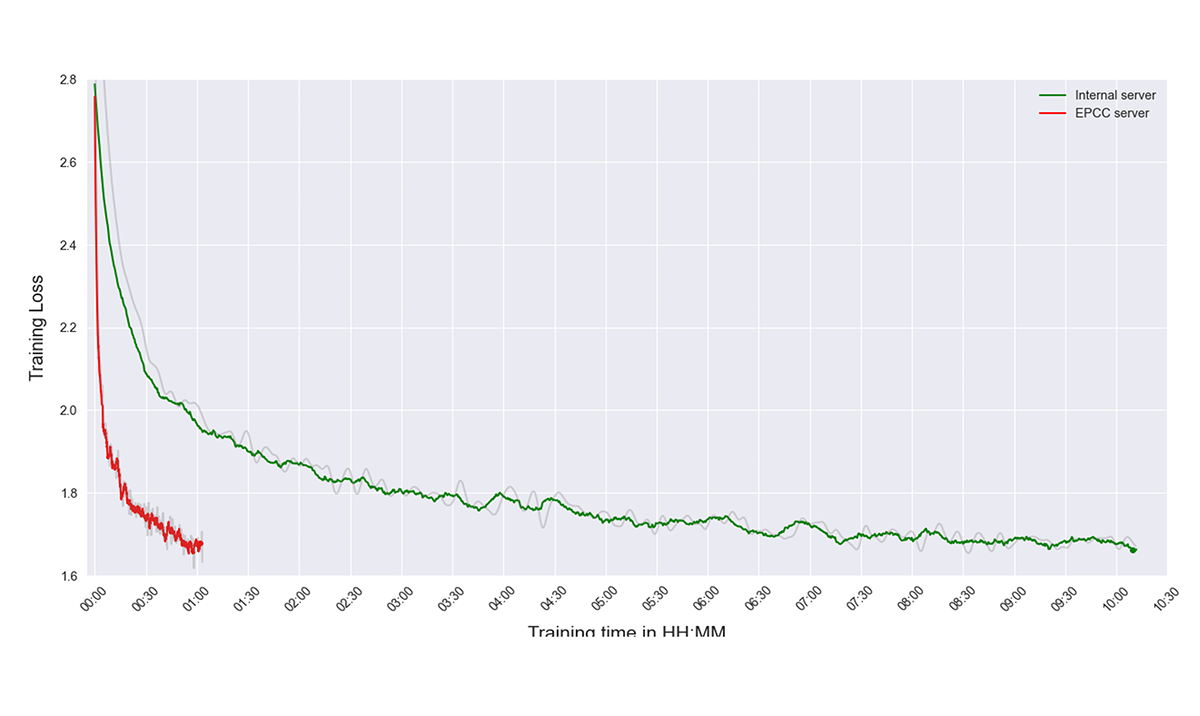
Oliver King-Smith Founder and CEO of smartR AI“We are very fortunate to be able to work with EPCC on this important LLM and GPT related performance project, and look forward to the potential to incorporate other similar tests with, for example, EPCC’s new Graphcore POD64 system."
Links
The Cerebras CS-2 service at EPCC is available through the Edinburgh International Data Facility, which we operate on behalf of the Data-Driven Innovation initiative.
To discuss how our supercomputing and data services can support your business goals, please contact Julien Sindt, EPCC's Commercial Manager: j.sindt@epcc.ed.ac.uk