PhD research: Affective dynamic model-based machine learning for cognitive emotion recognition
22 September 2023
Xingran Ruan, a PhD student based at EPCC, is investigating the recognition of cognitive emotions, including confusion, frustration, and boredom, with the aim of enhancing future Intelligent Tutor Systems (ITS) to provide targeted assistance. Here he describes his research.
Emotions are an integral part of our daily lives, influencing our experiences at work, during learning, and in various other situations. Have you ever found yourself feeling confused when faced with a challenging obstacle that tests your knowledge? Or perhaps you've experienced frustration when struggling to overcome such obstacles? Maybe you've even grown bored with your reading material after repeatedly attempting to overcome these hurdles.
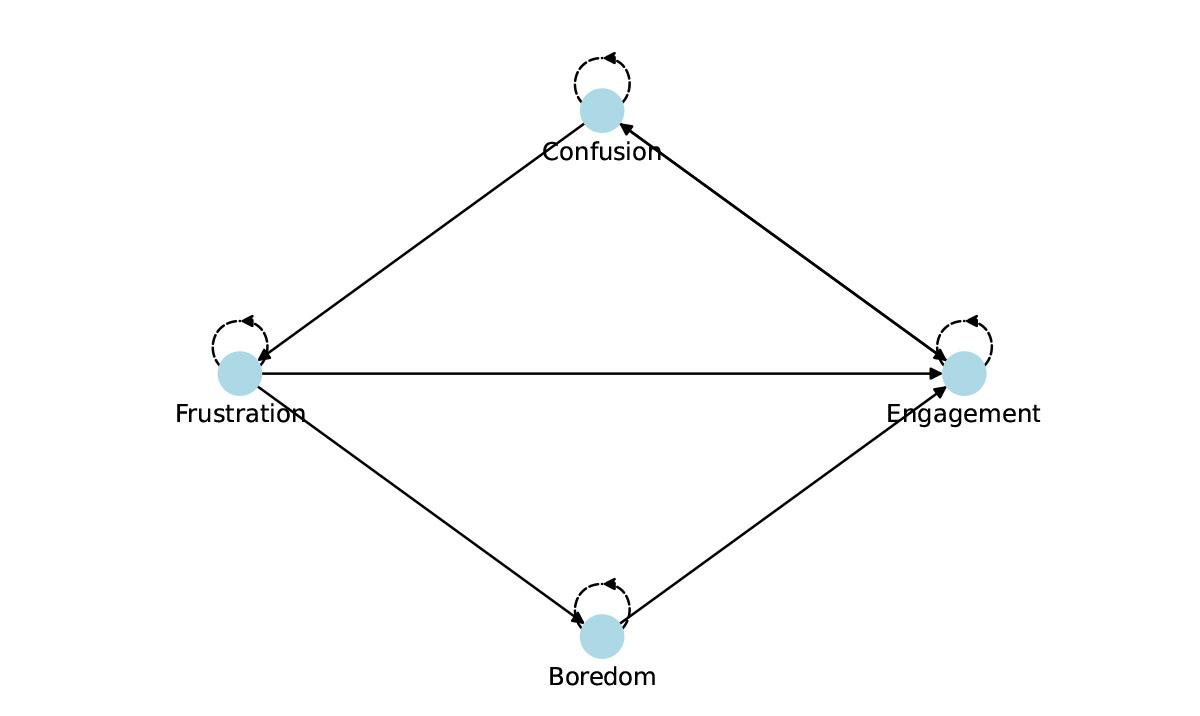
Why is Facial Emotion Recognition (FER) crucial for ITS?
Intelligent tutor systems (ITS) are computer-based tools designed to offer instant and tailored guidance to learners. Essentially, ITS strives to deliver individualised, one-on-one tutoring experiences for students as opposed to the traditional one-size-fits-all approach of classroom teaching.
Traditional ITSs have limitations; they can only offer support based on explicit indicators from learners, such as final scores or feedback. These systems often struggle to gauge a learner's true understanding of the material. Emotions serve as a window into a learner's cognitive processes. Emotions like boredom, confusion, frustration, and curiosity can be utilized to predict a learner's progress, and some cognitive emotions, such as confusion and boredom, can offer insights into metacognition—the process of thinking about one's own thinking. Thus FER techniques are important for ITS.
Our contributions to the research field
Emotion recognition is a prominent area within machine learning. Currently, numerous large-scale emotional datasets are available for pre-training FER models, including datasets like RAF-DB, AffectNet, and FER2013. While these datasets have significantly improved the performance of FER models, they primarily encompass a narrow range of basic emotions, such as anger, contempt, disgust, fear, happiness, sadness, and surprise. These emotions do not effectively capture the intricacies of the learning process. Furthermore, datasets focused on cognitive emotions are both limited in size and imbalanced, which can lead to overfitting issues during FER model training. Our work aims to addresses this overfitting challenge when working with small, imbalanced emotion datasets.
We have incorporated psychological aspects to a neural networks based machine learning model. The psychological model highlights that when learners come across challenges, their level of engagement can shift towards confusion. If these challenges persist without resolution, this initial confusion can develop into frustration over time. Eventually, if this frustration persists, it may lead to a state of boredom. You can refer to Figure 1 for a visual representation of this affective dynamic (AD) model.
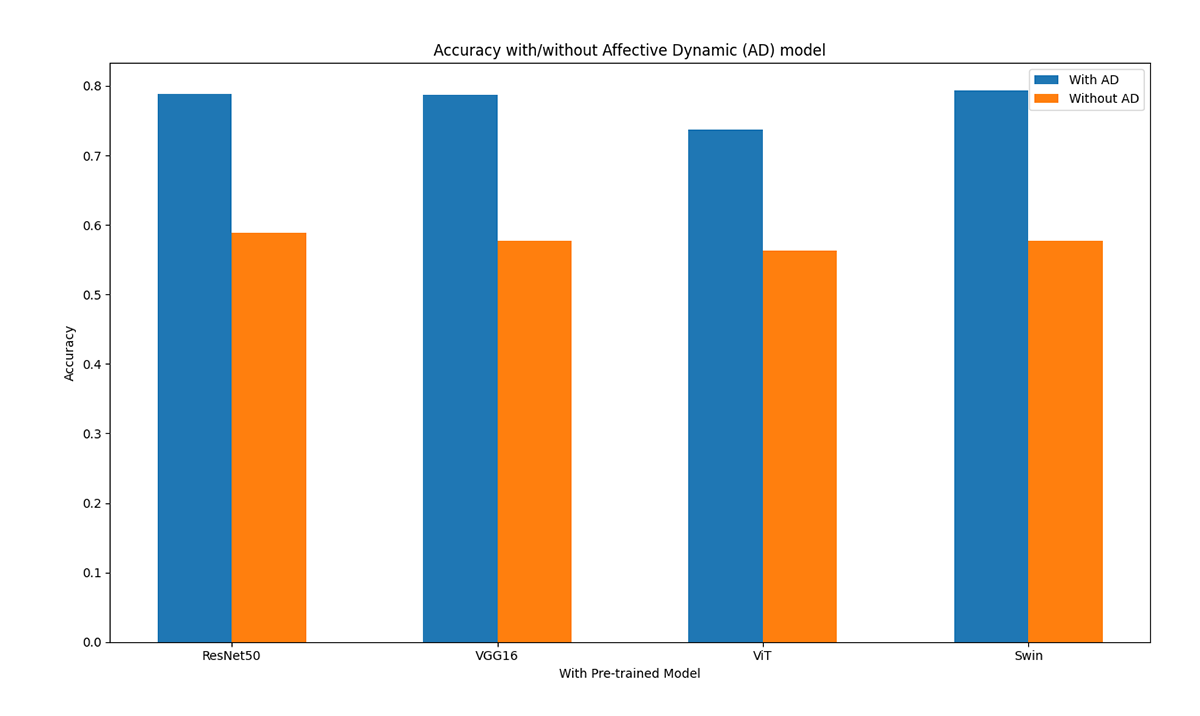
Our primary objective is to fine-tune weights within neural networks to replicate the behavior of the AD model in making predictions. To illustrate the impact of this, we have included a chart in Figure 2 that compares the performance of the network with AD to that without AD. Additionally, in Figure 3, we present the weight values of the network throughout the training process. This visualisation clearly illustrates that in the network without the AD model's calibration, a significant portion of the neurons remains 'inactive' (with no weight updates). In contrast, in the network incorporating the AD model, all neurons receive updates during training, highlighting the effectiveness of our approach.
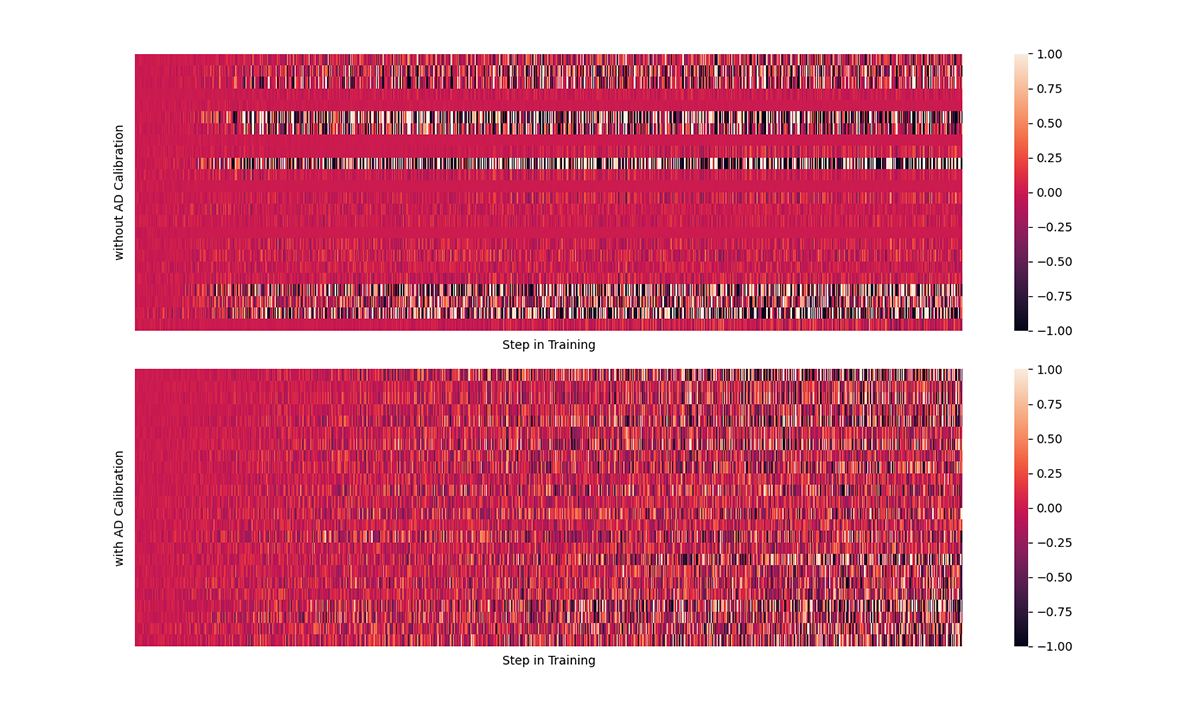
Our proposed method enables significantly accurate way of detecting emotions and transition between emotions for ITSs and then allowing ITSs to provide effective help with the learning process.
Authors
Xingran Ruan: xruan@ed.ac.uk
Charaka Palansuriya: charaka@epcc.ed.ac.uk
Aurora Constantin: aurora.constantin@ed.ac.uk
Paper presentation
Xingran presented his paper "Affective Dynamic based Technique for Facial Emotion Recognition (FER) to Support Intelligent Tutors in Education" at the 24th International Conference on Artificial Intelligence in Education (AIED Tokyo 23), Tokyo, Japan in July 2023.