Quantifying uncertainties in direct numerical simulations of a turbulent channel flow
25 June 2024
EPCC Chancellor's Fellow Joe O'Connor describes his recent research, which is of direct value to the turbulence modelling and simulation community.
Computational fluid dynamics (CFD) is the use of numerical modelling and computational simulation to study fluid mechanics. Direct numerical simulation (DNS) – where all spatial and temporal scales of the fluid are fully resolved – is the gold standard of CFD methods for turbulent flows. However, like all numerical methods, DNS is subject to model uncertainties arising from the numerical scheme and input parameters (eg mesh size). While uncertainty quantification (UQ) techniques are being employed more and more to provide a systematic analysis of uncertainties in lower-fidelity models, their application to DNS is extremely rare. This is primarily due to computational cost, with most UQ workflows requiring hundreds/thousands of model evaluations.
Considering this, the aim of this work was to, for the first time, apply rigorous UQ techniques to DNS of a canonical wall-bounded turbulent channel flow (Figure 1) in order to propagate uncertainties and assess the sensitivities of various quantities of interest (QOIs) with respect to certain inputs. To compute the DNS, we used Incompact3d – a highly scalable open-source framework based on high-order compact finite differences and a spectral Poisson solver – as a black box. To facilitate the non-intrusive forward UQ analysis, we used the EasyVVUQ Python library, developed by our collaborators at University College London and Centrum Wiskunde & Informatica (among others), which enables automated sampling, preprocessing, execution, postprocessing and UQ analysis on high-performance computing (HPC) platforms.
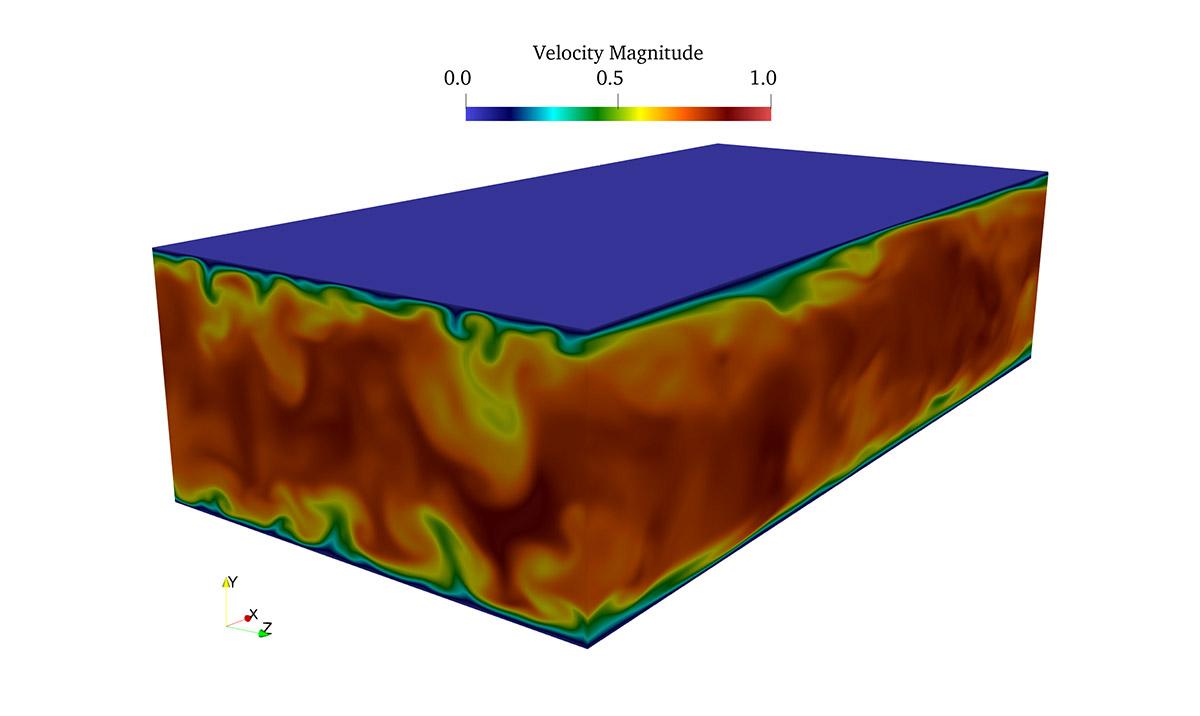
Figure 1: Illustrative snapshot of the turbulent channel flow case.
Uncertainty quantification campaigns
We performed three separate UQ campaigns. The first two campaigns used a standard sampling approach, based on Gaussian quadrature rules combined with tensor products, to sample the uncertain input space. For Campaign I, the input space considered just the size of the domain, whereas Campaign II considered just the numerical parameters (mesh size in each direction, time step size, sampling time) as the uncertain inputs. Overall, this required 25 (Campaign I) and 3125 (Campaign II) separate DNS evaluations, with each campaign requiring about 500,000 core-hours. The results show that the uncertainties mainly manifest in the second-order velocity moments (Figure 2) and that the sensitivity to a given input is highly dependent on the QOI.
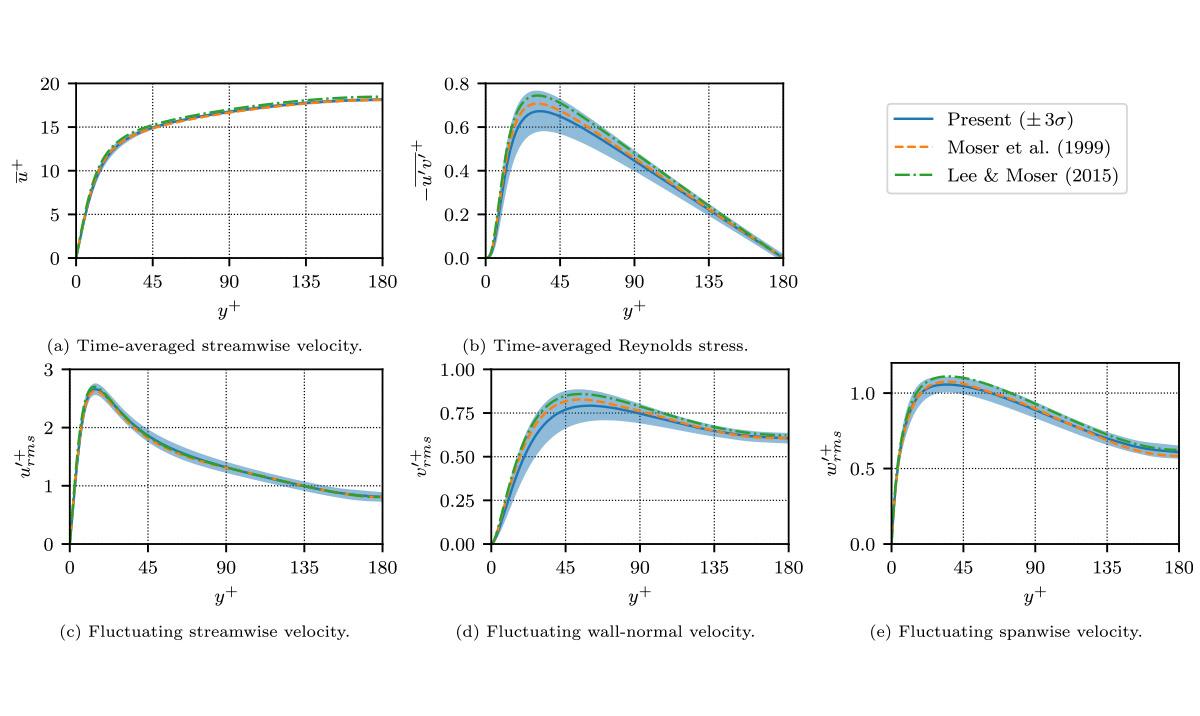
Figure 2: Cross-channel profiles of first and second-order velocity moments for Campaign II.
While these results were interesting and informative, they were expensive to obtain (approximately 1 million core-hours in total) and thus it is not feasible to incorporate such analyses into a typical CFD study. Therefore, the second phase of this work investigated the use of alternative “smart” sampling strategies to reduce the number of required model (DNS) evaluations. Here we adopted a dimension-adaptive sparse grid sampling strategy, which dynamically calculates and refines along the most “important” parameters in the input space.
For Campaign III, we applied this strategy to reproduce the results of Campaign II and assess the performance of the dimension-adaptive sparse grid strategy. The results show reasonably good agreement with the full tensor grid approach, although there is some difference in the observed variance (Figure 3). However, compared to the full tensor grid approach, which required 3125 separate DNS (500,000 core-hours), the dimension-adaptive sparse grid sampling strategy required just 37 separate DNS (14,00 core-hours). This level of compute effort is much more feasible and could be much more readily incorporated into a typical CFD workflow.
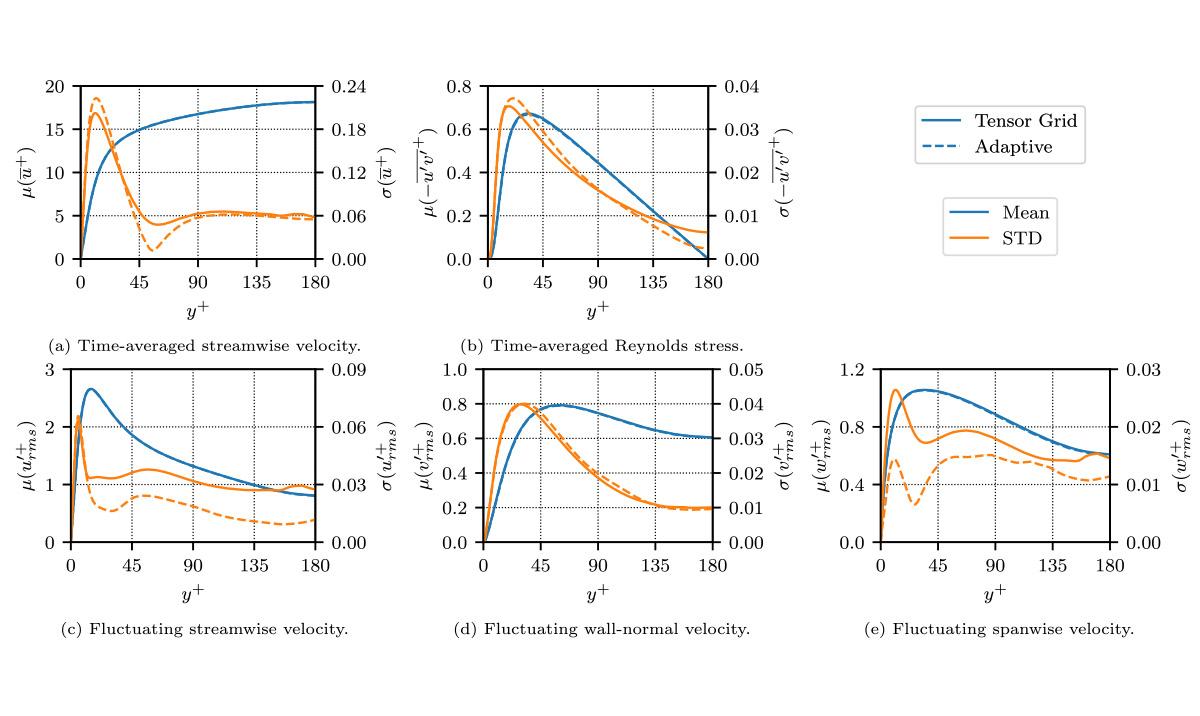
Figure 3: Comparison of cross-channel profiles of first and second-order velocity moments for the tensor grid and dimension-adaptive sampling schemes.
This work is useful to the turbulence modelling and simulation community as it demonstrates the application of UQ to DNS and highlights specific uncertainties/sensitivities associated with DNS of wall-bounded turbulence. However, this work is also useful to the wider modelling and simulation community as it demonstrates the potential benefits of adopting a “smart” sampling strategy to significantly reduce the compute effort required to perform these types of analyses. Ultimately, this should promote more widespread adoption of UQ techniques within the modelling and simulation community.
All of this work was enabled by HPC platforms provided by EPCC. In particular, both Cirrus and ARCHER2 were used to perform the Incompact3d simulations (DNS).
Link
Paper "Quantifying uncertainties in direct numerical simulations of a turbulent channel flow": https://www.sciencedirect.com/science/article/pii/S004579302300333X